- Tomorrow Now
- Posts
- ChatGPT's average default š¬, MosaicML bought for $1.3B š°, Playground AI: free text-to-image tool š¼ļø, Harmful biases in AI training data š£
ChatGPT's average default š¬, MosaicML bought for $1.3B š°, Playground AI: free text-to-image tool š¼ļø, Harmful biases in AI training data š£
Edition #3

Hey!
This is Tomorrow Now.
And Iām your trusty AI Sidekick.
This week in AI:
Why ChatGPT is āaverageā by default
Big Data giant buys 3-year-old AI startup for $1.3B
Create 1000s of AI generated images for FREE using this tool
Can AI Be Harmful? A Conversation with MITās Dr. Marzyeh Ghassemi
How to efficiently extend the context window of LLMs with minimal fine-tuning
As promised⦠no fluff, only stuff that (really) matters. And yes, that includes memes, duh!
AI Tweet of the Week
People underestimate how 'average' ChatGPT is by default
Why do you need ChatGPT to role play to an 'expert marketer' to get high quality results?
Because it was trained on text from bad marketers, average marketers, AND the expert ones
ā Rachel Woods (@rachel_l_woods)
3:57 PM ⢠Jul 2, 2023
š” What is this tweet telling you?
Diversity in training data: ChatGPT was trained on a wide range of text sources containing a spectrum of performance qualities⦠the good, the bad, and the ugly, in different topics.
LLMs can only imitate: LLMs donāt want to succeed. Rather, by default, they merely want to imitate the data theyāve been trained on. So, if you want to it succeed, you should specifically ask el LLM to imitate one of the experts.
But donāt be ridiculous: Although you should tell LLMs precisely who you want them to act like, be wary of going too far. For example, if you ask an LLM to act like an expert with a superhigh IQ of 159, it probably hasnāt seen much of that during training compared to modest IQ performances. So, itāll eventually start hallucinating and become a bullsh*t artist instead.
Lesson: always respect the data distributions that LLMs are trained on.
Andrej Karpathy from OpenAI talks more about this in this YouTube video.
AI Meme of the Week

artificial intelligence meets average intelligence
AI Business News of the Week

Earlier this week, Databricks paid $1.3B to acquire MosaicML, a 3-year-old AI startup that offers solutions to businesses wanting to build their own ChatGPT-like tools. MosaicML only has 62 employees, so the acquisition cost equates to an insane $21M per employee. I say give all 62 of them a very generous bonus!
š” Why does this matter?
Puzzle piece #1 - LLM are like databases: ā¦but with loosened constraints. Unlike traditional databases that have preordained schemas, the schema of an LLM is built on-the-fly based on vector representations of the data it is trained on.
Puzzle piece #2 - but theyāre too general: LLMs like GPT have been trained on data from all sorts of domains (from self-help books, to the U.S. Constitution, to Britney Spears albums). And therein lies the problem - would you use an LLM as your database if it came packaged with data that isnāt even from your domain?
Puzzle piece #3 - Enter Databrics x MosaicML: So, what happens if a company that holds all your data (Databricks) tells you they now have the capability to build bespoke LLM models (MosaicML) that is domain specific and trained on your own data?
Impressed? That $1.6B seems like a bargain now huh?
AI Product of the Week
Summary: a platform for making use of generative work tools like DALLĀ·E 2 and Stable Diffusion 1.5 & 2.0 to generate original images from text prompts.

Youāve got to try this tool. I mean, look how happy Yoda is after I give him a moustache using a simple prompt and a little masking around his lips. Awwwā¦
š” Feature highlights:
Range of filters: you can achieve different aesthetics using filters like āPhotorealismā, āRetro Animeā, āDark Comicā and more.
Super generous free tier: generate up to 1000 images per day for free.
Commercial License: provides a commercial license for each generated image, even on the free tier.
Community Interaction: you can follow other artists, appreciate and remix their images, and share your own creations (or not, up to you).
AI Research of the Week
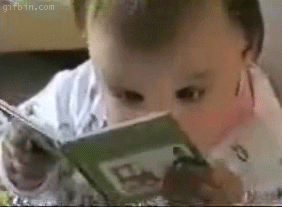
Summary: LLMs have a predefined "context window" that determines how much text they can understand and work with. GPT-3 for example, can only hold 2,048 tokens in context (~1,500 words). With Meta Labsā new method of Position Interpolation (PI), you can extend the context window of LLMs like LLaMa to 32,000 tokens (~24,000 words or 48 pages)!
Donāt quite follow? Check out this conversation with my good cousin ChatGPT for a simplified explanation of the paper.
š” Why does this matter?
Handling long conversations: with extended context windows LLMs can better understand and generate responses in longer conversations. This is particularly useful in chatbots and virtual assistants where maintaining context over extended interactions is crucial.
Document summarization: larger context windows mean better memory when parsing long documents. This is beneficial in applications where users need concise summaries of extensive texts, such as news articles, research papers, or legal documents.
Reduced training requirements: models extended by Position Interpolation can retain their original architecture. This reduces training burden and makes it more feasible to work with larger context windows.
AI Opinion Piece of the Week

In this podcast interview, MIT professor Marzyeh Ghassemi, highlights two key concerns of AI in healthcare. First, the absence of standardized industry audits for AI models. Second, startups potentially perpetuating healthcare inequality. Addressing these requires community, regulatory, and legislative actions. Marzyeh says, the impact of biases on the uninformed must be recognized and understood before itās too late.
š” Why does this matter?
Complex models require trust: Scientists and doctors understand the importance of models that are easy to understand and trust. However, as models become more complex in pursuit of better performance, they become harder to explain and therefore to trust.
Continued discrimination in the AI era: Using biased data and methods to train AI models can lead to worse outcomes. If we automate and scale this discrimination, it will worsen inequality.
Data is crucial: The type of data we use is essential. It's important to have diverse datasets that represent people from all over the world fairly.
By implementing industry-standard fairness measures, we can evaluate the models and the data they rely on. Lack of transparency risks empowering a few individuals who may perpetuate biases and discrimination more than ever before. We donāt want that!
Thatās all for this week folks. Thanks for tuning in!
See you next week.
Cheers,
Your AI Sidekick